TechBlog
[Kaggle/python] PUBG ๋ฐฐํ๊ทธ๋ผ์ด๋ ๊ฒ์ ๋ฐ์ดํฐ ๋ถ์ ๋ณธ๋ฌธ
[Kaggle/python] PUBG ๋ฐฐํ๊ทธ๋ผ์ด๋ ๊ฒ์ ๋ฐ์ดํฐ ๋ถ์
jiazzang 2023. 10. 10. 23:31
๐ ์ฃผ์
PUBG ๋ฐฐํ๊ทธ๋ผ์ด๋ ๊ฒ์ ๋ฐ์ดํฐ ๋ถ์
๐ ์์
1. ์ฃผ์ ์ ์
2. ๊ฒ์ ์ค๋ช
3. ํ์ฉ ๋ฐ์ดํฐ ๋ฐ ๋ณ์
4. ์๋ฃ ๋ถ์ ๊ณผ์
1. ์ฃผ์ ์ ์
- ์ฃผ์ : PUBG ๋ฐฐํ๊ทธ๋ผ์ด๋ ๊ฒ์ ๋ฐ์ดํฐ ๋ถ์
- ์์ฝ: PUBG Developer์์ ๊ณต๊ฐํ ๋ฐฐํ๊ทธ๋ผ์ด๋ ๋ฐ์ดํฐ๋ฅผ ํ์ฉํ ๋ถ์ ํ๋ก์ ํธ๋ฅผ ์งํํ์ต๋๋ค.
- ๋ถ์ ๋ด์ฉ: EDA, ์ ์ฒ๋ฆฌ, ์๊ฐํ๋ฅผ ์์ฃผ๋ก ๋ถ์์ ์งํํ๊ณ , ํ๋ ์ด์ด ๋ฑ๊ธ์ ์ต์ข ๋ฐฐ์น๋ฅผ ์์ธกํ๋ RandomForest ๋ชจ๋ธ๋ basicํ๊ฒ ๋ง๋ค์ด๋ณด์์ต๋๋ค.
2. ๊ฒ์ ์ค๋ช
๐ ๊ฒ์ ๊ฐ์
๋ฐฐํ๊ทธ๋ผ์ด๋๋ ํ๋ ์ด์ด๊ฐ ๋์๋ค๋๋ฉด์ ๋ฌด๊ธฐ, ์ด์, ๋ฐฉ์ด๊ตฌ, ๊ตฌ๊ธ์ฝ ๋ฑ์ ์์งํ๊ณ ์๋ก ์ฃฝ์ด๋ฉฐ ์ตํ๊น์ง ์ด์๋จ๋ ๊ฒ์์ ๋๋ค. ๊ฒ์ ์์ ์ ํ๋ ์ด์ด๋ค์ ๋นํ๊ธฐ์ ํ ์ํ์ด๋ฉฐ, ๊ฐ์ ์ํ๋ ์์น์ ๋ํํ ํ ํ๋ฐ(์์ดํ ์ ์ค๋ ํ์)์ ํตํด ์ธ์์ ์ํ ์ค๋น๋ฅผ ํฉ๋๋ค. ๊ฒ์์์ ์น๋ฆฌํ๊ธฐ ์ํ ์ ๋ต์ผ๋ก๋ ํฌ๊ฒ 1) ์๊ทน์ ์ ๋ต(๊ฑด๋ฌผ์ ์จ์ด์ ์ ์ ์ฃฝ์), 2) ์ ๊ทน์ ์ ๋ต(๋๊ฒฉ์ ํตํด ๋น ๋ฅด๊ฒ ์ ์ ์ฃฝ์)์ด ์์ต๋๋ค.
๐ท ๊ฒ์ ๊ท์น
- ๊ฐ ๊ฒฝ๊ธฐ(matchId)์๋ ์ต๋ 100๋ช ์ ํ๋ ์ด์ด๊ฐ ์ฐธ๊ฐํ ์ ์์ต๋๋ค.
- ํ ๊ฒฝ๊ธฐ ๋ด์์ ์ต๋ 4๋ช ์ ํ๋ ์ด์ด๊ฐ ๊ฐ์ ๊ทธ๋ฃน์ ํฌํจ๋ ์ ์์ต๋๋ค.
โ ๏ธ ์ฃผ์ํ ์
๋ฐ์ดํฐ ํ์ธ ๊ฒฐ๊ณผ, ํ ๊ฒฝ๊ธฐ ๋ด์์ groupId๊ฐ ๊ฐ์ ํ๋ ์ด์ด๊ฐ 4๋ช ์ด์์ธ ๊ฒฝ์ฐ๊ฐ ์กด์ฌํ์ต๋๋ค. Kaggle notebook์์ ์ฐธ๊ณ ํ ๋ฐ๋ก๋, ์ด๋ ๊ฒ์์์ ์ฐ๊ฒฐ์ด ๋๊ธฐ๊ธฐ ๋๋ฌธ์ ๋ฐ์ํ๋ ํ์์ด๋ผ๊ณ ํฉ๋๋ค. ์ฌ๋ฌ ๊ทธ๋ฃน์ ํ๋ ์ด์ด๊ฐ API์ ๋ฐ์ดํฐ๋ฒ ์ด์ค์ ๋์ผํ ์ต์ข ๋ฐฐ์น๋ฅผ ๊ฐ์ง ๊ฒ์ผ๋ก ์ ์ฅ๋๊ธฐ ๋๋ฌธ์ ๋๋ค. ๋ฐ๋ผ์ ์ฐ๋ฆฌ๋ groupId๋ฅผ ํ์คํ ํจ๊ปํ ํ์ด ์๋๋ผ ๋์ผํ ์ต์ข ์์๋ฅผ ๊ฐ์ง ํ๋ ์ด์ด๋ผ๊ณ ์๊ฐํด์ผ ํฉ๋๋ค.
2. ํ์ฉ ๋ฐ์ดํฐ ๋ฐ ๋ณ์
(1) ํ์ฉ ๋ฐ์ดํฐ
โถ train ๋ฐ์ดํฐ(.csv)
- Rows X Columns: 4446966 X 29
- ๊ฐ ํ์ ํ๋ ์ด์ด ํ ๋ช ์ ๊ฒ์(match) ํต๊ณ๋ฅผ ๋ํ๋
- Target variable: ํ๋ ์ด์ด ๋ฑ๊ธ์ ์ต์ข ๋ฐฐ์น(winPlacePerc)
(2) ํ์ฉ ๋ฐ์ดํฐ ์ถ์ฒ
โถ Kaggle - PUBG Finish Placement Prediction (Kernels Only)
(3) ํ์ฉ ๋ณ์
Data fields | Details |
DBNOs | Number of enemy players knocked |
assists | Number of enemy players this player damaged that were killed by teammates. |
boosts | Number of boost items used. |
damageDealt | Total damage dealt. Note: Self inflicted damage is subtracted. |
headshotKills | Number of enemy players killed with headshots. |
heals | Number of healing items used. |
Id | Player’s Id |
killPlace | Ranking in match of number of enemy players killed. |
killPoints | Kills-based external ranking of player. (Think of this as an Elo ranking where only kills matter.) If there is a value other than -1 in rankPoints, then any 0 in killPoints should be treated as a “None”. |
killStreaks | Max number of enemy players killed in a short amount of time. |
kills | Number of enemy players killed. |
longestKill | Longest distance between player and player killed at time of death. This may be misleading, as downing a player and driving away may lead to a large longestKill stat. |
matchDuration | Duration of match in seconds. |
matchId | ID to identify match. There are no matches that are in both the training and testing set. |
matchType | String identifying the game mode that the data comes from. The standard modes are “solo”, “duo”, “squad”, “solo-fpp”, “duo-fpp”, and “squad-fpp”; other modes are from events or custom matches. |
rankPoints | Elo-like ranking of player. This ranking is inconsistent and is being deprecated in the API’s next version, so use with caution. Value of -1 takes place of “None”. |
revives | Number of times this player revived teammates. |
rideDistance | Total distance traveled in vehicles measured in meters. |
roadKills | Number of kills while in a vehicle. |
swimDistance | Total distance traveled by swimming measured in meters. |
teamKills | Number of times this player killed a teammate. |
vehicleDestroy | Number of vehicles destroyed. |
walkDistance | Total distance traveled on foot measured in meters. |
weaponsAcquired | Number of weapons picked up. |
winPoints | Win-based external ranking of player. (Think of this as an Elo ranking where only winning matters.) If there is a value other than -1 in rankPoints, then any 0 in winPoints should be treated as a “None”. |
groupId | ID to identify a group within a match. If the same group of players plays in different matches, they will have a different groupId each time. |
numGroups | Number of groups we have data for in the match. |
maxPlace | Worst placement we have data for in the match. This may not match with numGroups, as sometimes the data skips over placements. |
winPlacePerc | The target of prediction. This is a percentile winning placement, where 1 corresponds to 1st place, and 0 corresponds to last place in the match. It is calculated off of maxPlace, not numGroups, so it is possible to have missing chunks in a match. |
4. ์๋ฃ ๋ถ์ ๊ณผ์
(1) ๊ธฐ๋ณธ ์ธํ
โก ํ์ํ ๋ผ์ด๋ธ๋ฌ๋ฆฌ ๋ถ๋ฌ์ค๊ธฐ
import numpy as np
import pandas as pd
import seaborn as sns
import matplotlib.pyplot as plt
%matplotlib inline
plt.rcParams['axes.unicode_minus'] = False
plt.rcParams['font.family'] = "Malgun Gothic"
plt.rcParams['axes.grid'] = False
color = sns.color_palette()
plt.style.use("fivethirtyeight")
import matplotlib.font_manager as fm
parameters = {'axes.labelsize': 10,
'axes.titlesize': 15,
'figure.titlesize': 17,
'xtick.labelsize': 11,
'ytick.labelsize': 14,
'legend.fontsize': 12,
'legend.title_fontsize': 13}
plt.rcParams.update(parameters)
import warnings
warnings.filterwarnings('ignore')
(2) ๋ฐ์ดํฐ ์ดํด๋ณด๊ธฐ
โก ๋ฐ์ดํฐ ๋ถ๋ฌ์ค๊ธฐ
## train ๋ฐ์ดํฐ ๋ก๋
train = pd.read_csv("./PUBG_train_V2.csv")
โก ๋ฐ์ดํฐ ํ์ธํ๊ธฐ
## ๋ฐ์ดํฐ ํ์ธ
train.head()
โก ํ, ์ด ๊ฐ์์ ๊ฒฐ์ธก์น ์กด์ฌ ์ฌ๋ถ ํ์ธํ๊ธฐ
## ํ/์ด ํ์ธ
print(train.shape)
# ์ปฌ๋ผ๋ณ ๊ฒฐ์ธก์น ๊ฐ์ ํ์ธ
print(train.isnull().sum())
# ์ถ๋ ฅ ๊ฒฐ๊ณผ
(4446966, 29)
Id 0
groupId 0
matchId 0
assists 0
boosts 0
damageDealt 0
DBNOs 0
headshotKills 0
heals 0
killPlace 0
killPoints 0
kills 0
killStreaks 0
longestKill 0
matchDuration 0
matchType 0
maxPlace 0
numGroups 0
rankPoints 0
revives 0
rideDistance 0
roadKills 0
swimDistance 0
teamKills 0
vehicleDestroys 0
walkDistance 0
weaponsAcquired 0
winPoints 0
winPlacePerc 1
dtype: int64
โก ๋ฉ๋ชจ๋ฆฌ ์ต์ ํํ๊ธฐ
## ๋ฉ๋ชจ๋ฆฌ ์ต์ ํํ๊ธฐ
# Memory saving function credit to https://www.kaggle.com/gemartin/load-data-reduce-memory-usage
def reduce_mem_usage(df):
""" iterate through all the columns of a dataframe and modify the data type
to reduce memory usage.
"""
#start_mem = df.memory_usage().sum() / 1024**2
#print('Memory usage of dataframe is {:.2f} MB'.format(start_mem))
for col in df.columns:
col_type = df[col].dtype
if col_type != object:
c_min = df[col].min()
c_max = df[col].max()
if str(col_type)[:3] == 'int':
if c_min > np.iinfo(np.int8).min and c_max < np.iinfo(np.int8).max:
df[col] = df[col].astype(np.int8)
elif c_min > np.iinfo(np.int16).min and c_max < np.iinfo(np.int16).max:
df[col] = df[col].astype(np.int16)
elif c_min > np.iinfo(np.int32).min and c_max < np.iinfo(np.int32).max:
df[col] = df[col].astype(np.int32)
elif c_min > np.iinfo(np.int64).min and c_max < np.iinfo(np.int64).max:
df[col] = df[col].astype(np.int64)
else:
if c_min > np.finfo(np.float16).min and c_max < np.finfo(np.float16).max:
df[col] = df[col].astype(np.float16)
elif c_min > np.finfo(np.float32).min and c_max < np.finfo(np.float32).max:
df[col] = df[col].astype(np.float32)
else:
df[col] = df[col].astype(np.float64)
#end_mem = df.memory_usage().sum() / 1024**2
#print('Memory usage after optimization is: {:.2f} MB'.format(end_mem))
#print('Decreased by {:.1f}%'.format(100 * (start_mem - end_mem) / start_mem))
return df
train = reduce_mem_usage(train)
(3) ๋ถํฌ ํ์ธํ๊ธฐ
โก ๋ณ์๋ณ Plot
- ๋ณ์๋ณ Plot์ ํ์ธํด๋ณธ ๊ฒฐ๊ณผ, "Kill", "longestKill", "walkDistance", "rideDistance", "swimDistance" ๋ฑ target ๋ณ์์ ์ ์๋ฏธํ ์ํฅ์ ์ค ๊ฒ์ผ๋ก ์์๋๋ ๋ณ์๋ค์ ๋ถํฌ ๋ถ๊ท ํ์ด ์ฌํ ๊ฒ์ผ๋ก ํ๋จ๋จ
- ๋ฐ๋ผ์ ํด๋น ๋ณ์๋ค์ ๋ํด ์ด์์น ์ ๊ฑฐ ํ์
โ๏ธ ํ๋ ์ด์ด๋ค์ Kill ํ์ ๋ถํฌ
## ํ๋ ์ด์ด๋ค์ Kill ํ์ ๋ถํฌ
plt.figure(figsize=(12,4))
sns.countplot(x='kills', data=train)
plt.title('ํ๋ ์ด์ด๋ค์ kills ํ์')
plt.show()
print("\n", "kills ํ์ ํ๊ท :", train['kills'].mean())
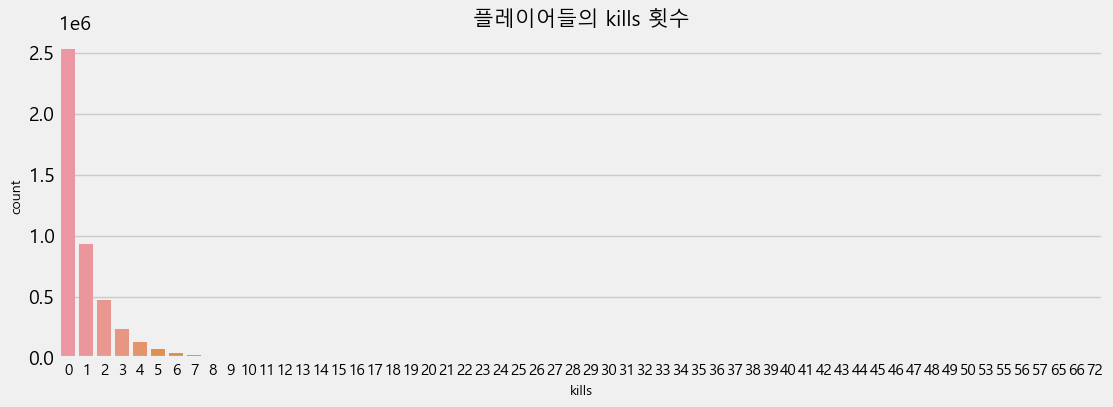
kills ํ์ ํ๊ท : 0.9247833241810259
ํด์
- ๋๋ถ๋ถ์ ํ๋ ์ด์ด๋ค์ 0ํฌ์ด๊ณ , ์ ๊ฒ๋ 1ํฌ๋ถํฐ ๋ง๊ฒ๋ 72ํฌ๊น์ง ๋๊ฒ ๋ถํฌํจ
โ๏ธ ํ๋ ์ด์ด๋ค์ matchDuration ๋ถํฌ
## ํ๋ ์ด์ด๋ค์ matchDuration(๋งค์น์ง์์๊ฐ) ๋ถํฌ
plt.figure(figsize=(12,4))
sns.distplot(x=train['matchDuration'], bins=10)
plt.title('ํ๋ ์ด์ด๋ค์ matchDuration ๋ถํฌ')
plt.show()
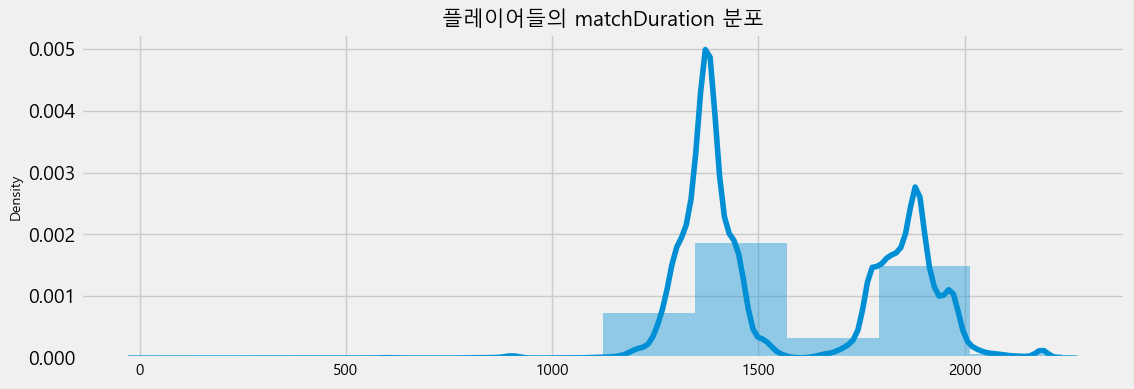
ํด์
- ๋๋ถ๋ถ์ ํ๋ ์ด์ด๋ 1000์ด ์ด์ ๋งค์น๋ฅผ ์ง์ํจ
โ๏ธ ํ๋ ์ด์ด๋ค์ teamKills ํ์ ๋ถํฌ
## ํ๋ ์ด์ด๋ค์ ํํฌ ํ์ ๋ถํฌ
plt.figure(figsize=(12,4))
sns.countplot(x='teamKills', data=train)
plt.title('ํ๋ ์ด์ด๋ค์ teamKills ํ์')
plt.ylim([0, 100000]) # y์ถ ๋ฒ์ ์ ํํด์ ๋ณด๊ธฐ
plt.show()
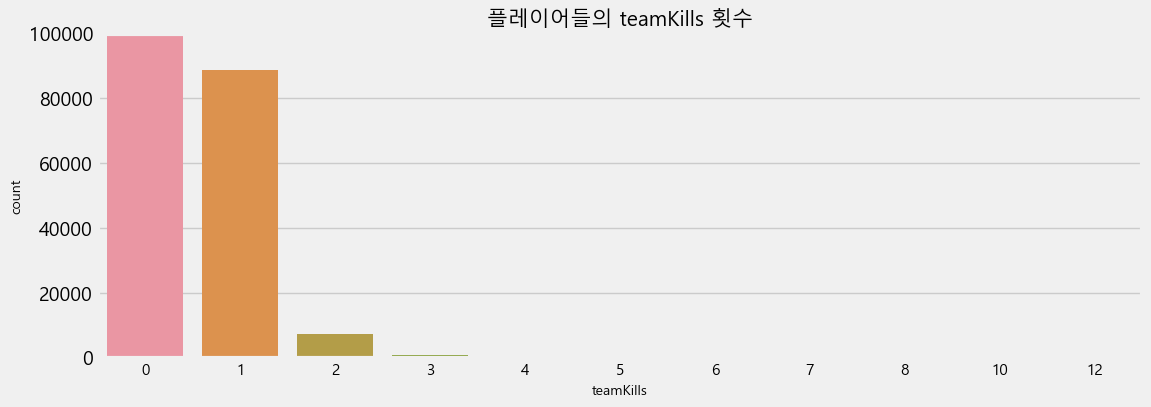
ํด์
- ๋๋ถ๋ถ์ ํ๋ ์ด์ด๋ค์ ํํฌ์ ํ์ง ์์ง๋ง, 1๋ฒํ๋ ๊ฒฝ์ฐ๊ฐ 8๋ง ๊ฑด ์ด์์
- ํํฌ์ ํ๋ ์ด์ ๋ ๋ค์๊ณผ ๊ฐ์ด ์ถ์ธกํด๋ณผ ์ ์์
- ๋จ์ํ ์ฌ๋ฏธ๋ฅผ ์ํด
- ํ์์ด ๋๋ฌด ๋ชปํด์ (์ฆ, ๊ฒ์์ ๋์์ด ๋์ง ์์ ๊ฒ ๊ฐ์์...)
โ๏ธ ํ๋ ์ด์ด๋ค์ longestKill ๋ถํฌ
## ํ๋ ์ด์ด๋ค์ longestKill ๋ถํฌ
plt.figure(figsize=(12,4))
sns.distplot(x=train['longestKill'], bins=10)
plt.title('ํ๋ ์ด์ด๋ค์ longestKill ๋ถํฌ')
plt.xlim([0, 200]) # x์ถ ๋ฒ์ ์ ํํด์ ๋ณด๊ธฐ
plt.show()
ํด์
- ๋๋ถ๋ถ 0~25m ์ด๋ด์ ๋ถํฌํ๊ณ ์์ผ๋, ๊ฐํน ์์ฃผ ๋จผ ๊ฑฐ๋ฆฌ์์ ์ฃฝ์ด๋ ๊ฒฝ์ฐ๋ ์กด์ฌํจ (์ด์์น ์ ๊ฑฐ ํ์)
โ๏ธ ํ๋ ์ด์ด๋ค์ walkDistance ๋ถํฌ
## ํ๋ ์ด์ด๋ค์ walkDistance ๋ถํฌ
plt.figure(figsize=(12,4))
sns.distplot(x=train['walkDistance'], bins=10)
plt.title('ํ๋ ์ด์ด๋ค์ walkDistance ๋ถํฌ')
plt.show()
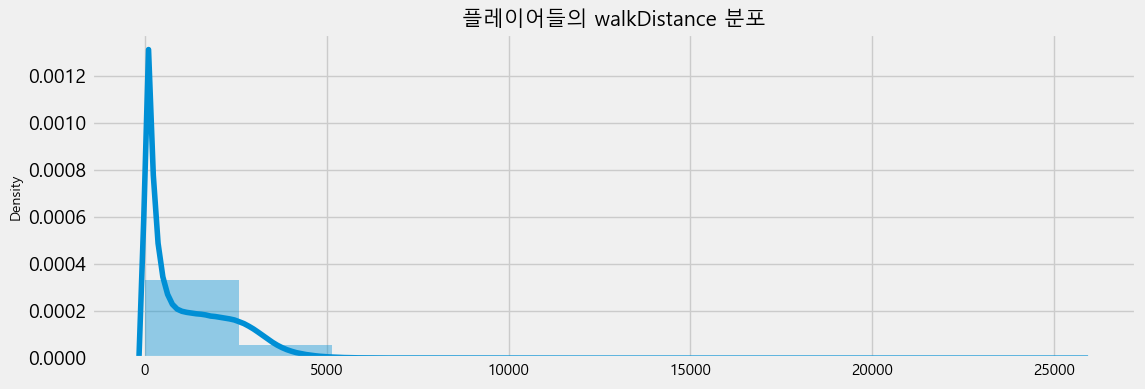
ํด์
- ๋๋ถ๋ถ์ ํ๋ ์ด์ด๋ค์ ํ ๋งค์น ๋ด์์ 0~2500m ์ ๋ ๋๋ณด๋ก ์ด๋ํ์ง๋ง, 25000m๋ฅผ ๋๋ ๊ฒฝ์ฐ๋ ์กด์ฌํจ (์ด์์น ์ ๊ฑฐ ํ์)
โ๏ธ ํ๋ ์ด์ด๋ค์ rideDistance ๋ถํฌ
## ํ๋ ์ด์ด๋ค์ rideDistance ๋ถํฌ
plt.figure(figsize=(12,4))
sns.distplot(x=train['rideDistance'], bins=10)
plt.title('ํ๋ ์ด์ด๋ค์ rideDistance ๋ถํฌ')
plt.show()
โ๏ธ ํ๋ ์ด์ด๋ค์ swimDistance ๋ถํฌ
## ํ๋ ์ด์ด๋ค์ swimDistance ๋ถํฌ
plt.figure(figsize=(12,4))
sns.distplot(x=train['swimDistance'], bins=10)
plt.title('ํ๋ ์ด์ด๋ค์ swimDistance ๋ถํฌ')
plt.show()
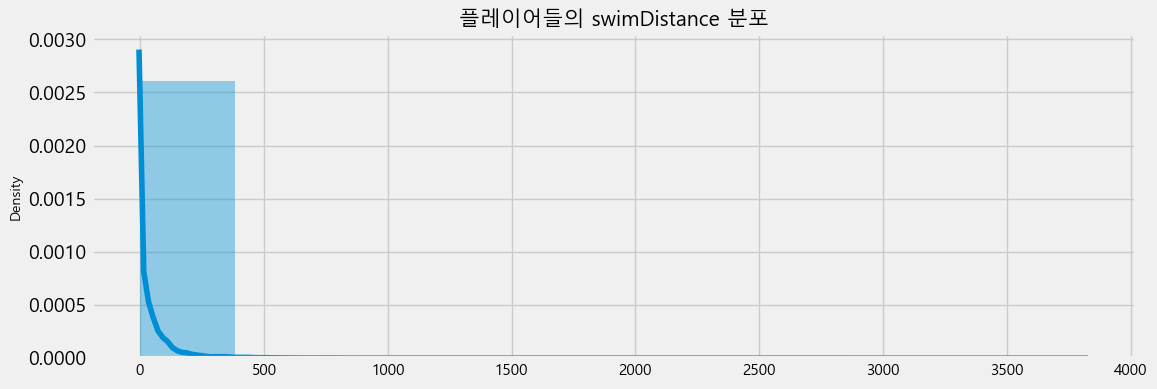
ํด์
- ๋๋ถ๋ถ์ ํ๋ ์ด์ด๋ค์ ์์์ ํตํด 0~500m ์ ๋ ์ด๋ํ์ง๋ง, 3500m ์ด์์ธ ๊ฒฝ์ฐ๋ ์กด์ฌํจ (์ด์์น ์ ๊ฑฐ ํ์)
โก ํ ๋งค์น๋น ๋ช ๋ช ์ ํ๋ ์ด์ด๊ฐ ์ฐธ๊ฐํ ๊น?
- ํ ๋งค์น์ 95~98๋ช ์ ํ๋ ์ด์ด๊ฐ ์ฐธ๊ฐํ๋ ๊ฒฝ์ฐ๊ฐ ๋๋ถ๋ถ์
- ๋งค์น๋ ๋๋ถ๋ถ ์ฐธ๊ฐ ๊ฐ๋ฅํ ์ต๋ ํ๋ ์ด์ด ์ 100๋ช ์ด ๊ฑฐ์ ์ฑ์์ ธ์ ์งํ๋๋ ํธ์
# "matchId"๋ณ๋ก playersJoined ๊ณ์ฐํ์ฌ ์๋ก์ด ์ปฌ๋ผ์ผ๋ก ์ถ๊ฐ
train['playersJoined'] = train.groupby('matchId')['matchId'].transform('count')
# ํ ๋งค์น์ 75๋ช
์ด์ ์ฐธ๊ฐํ ๊ฒฝ์ฐ๋ง ์๊ฐํ
plt.figure(figsize=(8,4))
sns.countplot(x=train[train['playersJoined']>=75]['playersJoined'])
plt.title('playersJoined')
plt.show()
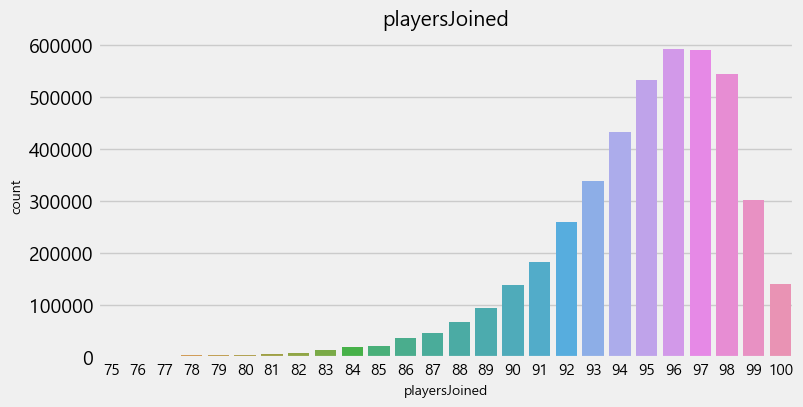
โก ์๋ก/๋์ค/์ค์ฟผ๋ ์ค ๊ฐ์ฅ ์น๋ฅ ์ด ๋์ ์ ํ์?
- ๋์ฒด์ ์ผ๋ก ์๋ก, ๋์ค, ์ค์ฟผ๋ ์์ผ๋ก ์น๋ฅ ์ด ๋์ ํธ์
# ๋งค์น ์ข
๋ฅ ํ์ธ
train['matchType'].value_counts()
# ์ถ๋ ฅ ๊ฒฐ๊ณผ
matchType
squad-fpp 1756186
duo-fpp 996691
squad 626526
solo-fpp 536762
duo 313591
solo 181943
normal-squad-fpp 17174
crashfpp 6287
normal-duo-fpp 5489
flaretpp 2505
normal-solo-fpp 1682
flarefpp 718
normal-squad 516
crashtpp 371
normal-solo 326
normal-duo 199
Name: count, dtype: int64
# "solo", "duo", "squad" ํค์๋๊ฐ ํฌํจ๋ ๋ฐ์ดํฐ๋ค๋ง ์ถ์ถ
solo = train[train['numGroups'] > 50]
duo = train[(train['numGroups'] > 25) & (train['numGroups'] <= 50)]
squad = train[train['numGroups'] <= 25]
# ๊ทธ๋ํ ์๊ฐํ
f,ax1 = plt.subplots(figsize=(20,10))
sns.pointplot(x='kills', y='winPlacePerc', data=solo, color='black')
sns.pointplot(x='kills', y='winPlacePerc', data=duo, color='#CC0000')
sns.pointplot(x='kills', y='winPlacePerc', data=squad, color='#3399FF')
plt.text(37,0.6, 'Solo', color='black', fontsize=17, style='italic')
plt.text(37,0.55, 'Duo', color='#CC0000', fontsize=17, style='italic')
plt.text(37,0.5, 'squad', color='#3399FF', fontsize=17, style='italic')
plt.xlabel('Number of kills', fontsize=15, color='blue')
plt.ylabel('Win Percentage', fontsize=15, color='blue')
plt.title('Solo vs Duo vs Squad Kills', fontsize=20, color='blue')
plt.grid() # ๊ทธ๋ฆฌ๋ ํ์
plt.show()
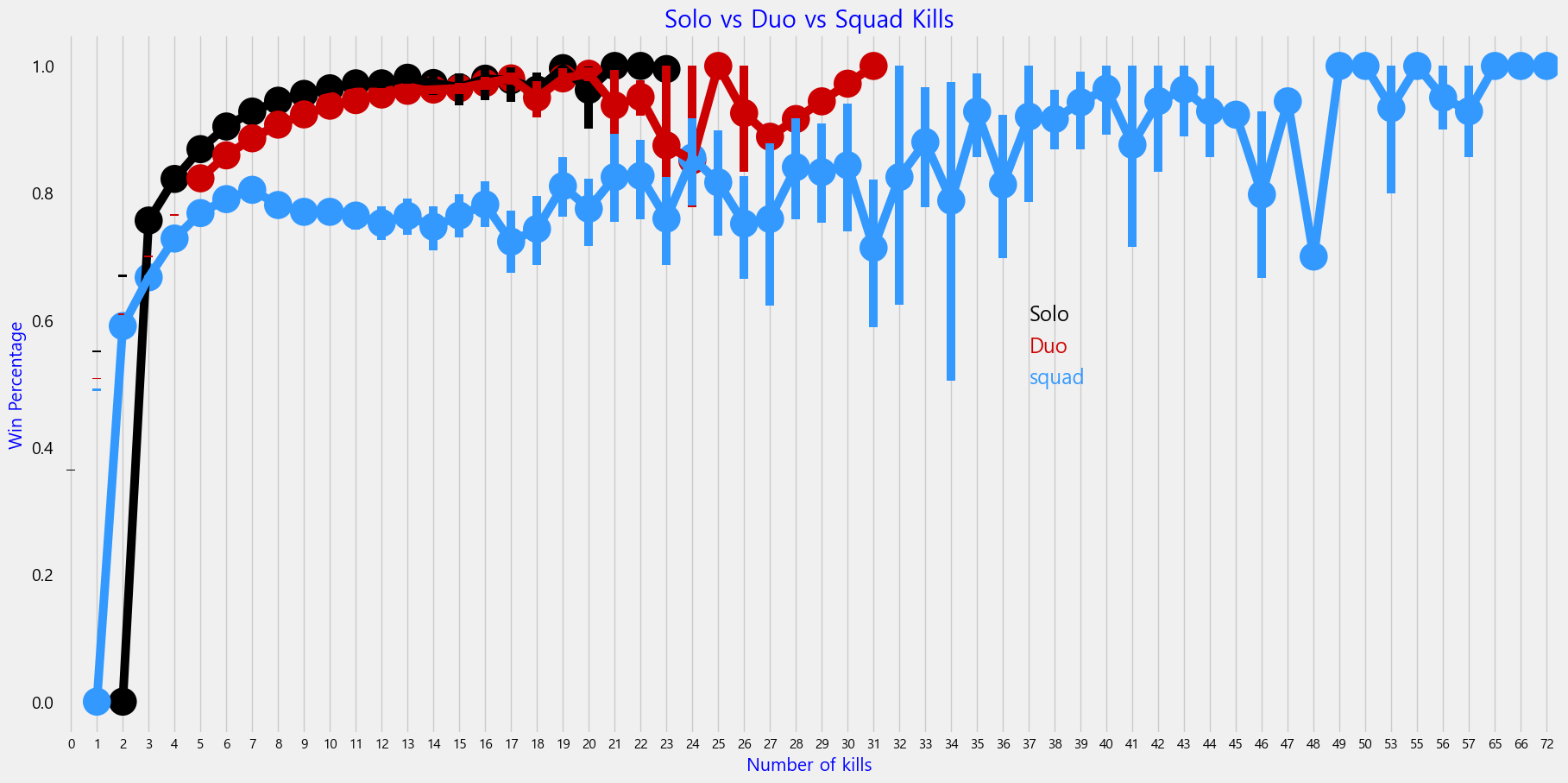
โก Kill์ ๋ง์ด ํ ์๋ก ์น๋ฅ ์ด ์ฌ๋ผ๊ฐ๊น?
- ๋๋ถ๋ถ์ ๊ฒฝ์ฐ Kill ์๊ฐ ๋ง์ ๋ ์น๋ฅ ๋ํ ๋์ ๊ฒ์ผ๋ก ๋ณด์
# kill ํ์์ ์น๋ฅ ์ ์ฐ์ ๋
plt.figure(figsize=(6,4))
plt.scatter(x = train['winPlacePerc'], y = train['kills'], color="red", alpha=0.3)
plt.title('kill ํ์์ ์น๋ฅ ์ฌ์ด์ ์ฐ์ ๋')
plt.show()
# kill ํ์ ๋ฒ์ฃผ๋ณ box plot
kills = train[['kills', 'winPlacePerc']]
kills['killsCategories'] = pd.cut(kills['kills'], [-1, 0, 2, 5, 10, 80], labels=['0 kills','1-2 kills', '3-5 kills', '6-10 kills', '10+ kills'])
plt.figure(figsize=(8,4))
sns.boxplot(x="killsCategories", y="winPlacePerc", data=kills)
plt.show()
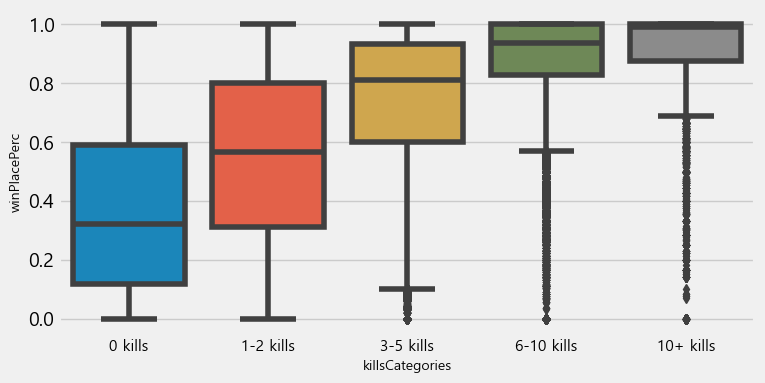
(4) ๋ฐ์ดํฐ ์ ์ฒ๋ฆฌ
โก ๊ฒฐ์ธก์น ์ฒ๋ฆฌ
- "winPlacePerc"์ ๊ฒฐ์ธก์น 1๊ฐ ์กด์ฌ => ํ์ธํด๋ณธ ๊ฒฐ๊ณผ, ์ค์ง ํ ๋ช ์ ํ๋ ์ด์ด๋ง ์ฐธ๊ฐํ ๊ฒฝ๊ธฐ์ธ ๊ฒ์ผ๋ก ํ๋จ๋๋ฏ๋ก ํด๋น ๋ฐ์ดํฐ ์ ๊ฑฐ
# "winPlacePerc"์ ๊ฒฐ์ธก์น 1๊ฐ ์กด์ฌ
print("๊ฒฐ์ธก์น ๊ฐ์:", train['winPlacePerc'].isnull().sum())
# ์ถ๋ ฅ ๊ฒฐ๊ณผ
๊ฒฐ์ธก์น ๊ฐ์: 1
# ๊ฒฐ์ธก์น๊ฐ ํฌํจ๋ ๋ฐ์ดํฐ ํ์ธ
train[train['winPlacePerc'].isnull()]
# ๊ฒฐ์ธก์น ํ ์ ๊ฑฐ ํ ์ธ๋ฑ์ค ์ฌ์ ๋ ฌ
train = train.drop(2744604).reset_index(drop=True)
โก ํ์๋ณ์ ์์ฑ
# ํ ํ์์ ๋ถ์คํธ ํ์๋ฅผ ๋ํ ํ์๋ณ์("healsandboosts") ์์ฑ
train['healsandboosts'] = train['heals'] + train['boosts']
# ๊ธฐ์กด๋ณ์("heals", "boosts") ์ ๊ฑฐ
train.drop(['heals', 'boosts'], axis=1, inplace=True)
โก ์ด์์น ํ์
- totalDistance: ํ๋ ์ด์ด๊ฐ ๊ฒ์ ์ค์ ํ ๋ฒ๋ ์์ง์ด์ง ์์ผ๋ฉด์ kill ํ์๊ฐ 1 ์ด์์ธ ๊ฒฝ์ฐ, ์ด์์น๋ก ํ๋จํ๊ณ ์ ๊ฑฐ
- roadKills: ๋ก๋ํฌ์ 10ํ ์ด์ ์ค์ํ ๊ฒฝ์ฐ, ์ด์์น๋ก ํ๋จํ๊ณ ์ ๊ฑฐ
- longestKill, walkDistance, rideDistance, swimDistance: ๊ฐ๊ฐ1km, 10km, 20km, 2km ์ด์์ด๋ฉด ์ด์์น๋ก ํ๋จํ๊ณ ์ ๊ฑฐ
## ํ ๋ฒ๋ ์์ง์ด์ง ์์ผ๋ฉด์ kill ํ์๊ฐ 1 ์ด์์ธ ๊ฒฝ์ฐ ์ด์์น๋ก ํ๋จ ํ ์ ๊ฑฐ
# ํ๋ ์ด์ด๊ฐ ์์ง์ธ ์ด ๊ฑฐ๋ฆฌ๋ฅผ ๋ํ๋ด๋ ๋ณ์ ์์ฑ ("totalDistance")
train['totalDistance'] = train['rideDistance'] + train['walkDistance'] + train['swimDistance']
# ์ด์์น ์ ๊ฑฐ
train['killsWithoutMoving'] = ((train['kills'] > 0) & (train['totalDistance'] == 0))
train.drop(train[train['killsWithoutMoving'] == True].index, inplace=True)
# ํ์ ์๋ ๋ณ์ ๋ชจ๋ ์ ๊ฑฐํด์ฃผ๊ธฐ
train.drop(['totalDistance', 'killsWithoutMoving'], axis=1, inplace=True)
## "roadKills"์ 10ํ ์ด์ ์ค์ํ ๊ฒฝ์ฐ ์ด์์น๋ก ํ๋จ ํ ์ ๊ฑฐ
train.drop(train[train['roadKills'] > 10].index, inplace=True)
## "longestKill"๊ฐ 1km ์ด์์ด๋ฉด ์ด์์น๋ก ํ๋จํ๊ณ ์ ๊ฑฐ
train.drop(train[train['longestKill'] >= 1000].index, inplace=True)
## Distance๊ฐ ๊ฐ๊ฐ 10km, 20km, 2km ์ด์์ด๋ฉด ์ด์์น๋ก ํ๋จํ๊ณ ์ ๊ฑฐ
train.drop(train[train['walkDistance'] >= 10000].index, inplace=True)
train.drop(train[train['rideDistance'] >= 20000].index, inplace=True)
train.drop(train[train['swimDistance'] >= 2000].index, inplace=True)
# ์ด์์น๋ฅผ ๋ชจ๋ ์ ๊ฑฐํ๊ณ ๋จ์ ๋ฐ์ดํฐ ๊ฐ์
train.shape
# ์ถ๋ ฅ ๊ฒฐ๊ณผ
(4445024, 29)
โก ๋ฒ์ฃผํ ๋ณ์ ์ธ์ฝ๋ฉ
- matchType: ์ํซ์ธ์ฝ๋ฉ
- groupId, matchId: categoryํ์ผ๋ก ๋ณํ (group๊ณผ match ๊ฐ ์๊ด๊ด๊ณ ํ์ฉ)
## "matchType": ์ํซ์ธ์ฝ๋ฉ
train = pd.get_dummies(train, columns=['matchType'])
# boolean(True/False)๋ฅผ int(0,1)๋ก ๋ณํ
train[train.columns[27:]] = train[train.columns[27:]].astype(int)
## "groupId", "matchId": categoryํ์ผ๋ก ๋ณํ ํ ์ซ์๊ฐ์ผ๋ก ์ธ์ฝ๋ฉ
train['groupId'] = train['groupId'].astype('category')
train['matchId'] = train['matchId'].astype('category')
train['groupId_cat'] = train['groupId'].cat.codes
train['matchId_cat'] = train['matchId'].cat.codes
# ๊ธฐ์กด ๋ณ์ ์ ๊ฑฐ
train.drop(['groupId', 'matchId'], axis=1, inplace=True)
# ์ธ์ฝ๋ฉ์ด ์ ๋์๋์ง ํ์ธ
train[['groupId_cat', 'matchId_cat']].head()
โก ํ์ ์๋ ๋ณ์ ์ ๊ฑฐ
## ํ๋ ์ด์ด์ ID ์ปฌ๋ผ ์ ๊ฑฐ
print("Id ๊ณ ์ ๊ฐ ๊ฐ์:", train['Id'].nunique())
train.drop("Id", axis=1, inplace=True)
# ์ถ๋ ฅ ๊ฒฐ๊ณผ
Id ๊ณ ์ ๊ฐ ๊ฐ์: 4445024
(5) ํ์ต ๋ฐ ํ๊ฐ
## ๋จธ์ ๋ฌ๋์ ์ํ ํจํค์ง
from sklearn.metrics import mean_absolute_error
from sklearn.ensemble import RandomForestRegressor
## ๋๋ฒ๊น
์ ์ํ ์ํ๋ง
sample = 500000
df_sample = train.sample(sample)
## target ๋ณ์ ๋ฐ๋ก ์ ์ฅ
y = df_sample['winPlacePerc']
df = df_sample.drop('winPlacePerc', axis=1)
โก ๊ฒ์ฆ์ ์ํ ๋ฐ์ดํฐ ๋ถ๋ฆฌ
## train, valid ๋ฐ์ดํฐ ๋ถ๋ฆฌํ๋ ํจ์ ์์ฑ
def split_vals(a, n:int):
return a[:n].copy(), a[n:].copy()
val_perc = 0.12
n_valid = int(val_perc * sample)
n_trn = len(df) - n_valid
# Split
raw_train, raw_valid = split_vals(df_sample, n_trn)
X_train, X_valid = split_vals(df, n_trn)
y_train, y_valid = split_vals(y, n_trn)
# ํ์ธ
print('train:', X_train.shape, 'target:', y_train.shape, 'validation:', X_valid.shape)
# ์ถ๋ ฅ ๊ฒฐ๊ณผ
train: (440000, 42) target: (440000,) validation: (60000, 42)
โก ํ๊ฐ ์งํ(MAE)
## ์ฑ๋ฅ ํ๊ฐ ์งํ(MAE) ์ถ๋ ฅํ๋ ํจ์ ์์ฑ
def print_score(m: RandomForestRegressor):
res = ['mae train:', mean_absolute_error(m.predict(X_train), y_train),
'mae val:', mean_absolute_error(m.predict(X_valid), y_valid)]
if hasattr(m, 'oob_score_'): res.append(m.oob_score_)
print(res)
โก Basic RF Model 1
## Basic model ํ์ต
rf = RandomForestRegressor(n_estimators=50,
min_samples_leaf=3,
max_features='sqrt',
n_jobs=-1)
rf.fit(X_train, y_train)
print_score(rf)
# ์ถ๋ ฅ ๊ฒฐ๊ณผ
['mae train:', 0.041673329186753184, 'mae val:', 0.06266519503048588]
โก Feature Importance
## Basic model์ ๋ณ์์ค์๋ ํ์ธ
rf_feature_importance = pd.DataFrame(rf.feature_importances_, X_train.columns, columns=['Feature Importance'])
# ๋ณ์์ค์๋ ์์๋ก ์ค๋ฆ์ฐจ์ ์ ๋ ฌ
rf_feature_importance = rf_feature_importance.sort_values('Feature Importance', ascending=False)
# ๋ณ์์ค์๋ ์๊ฐํ
plt.figure(figsize=(18,9))
sns.barplot(x='Feature Importance', y=rf_feature_importance.index, orient='h', data=rf_feature_importance)
plt.title("Feature Importance of RF", size=20)
plt.xticks(size=15)
plt.yticks(size=15)
plt.xlabel('feature importance', size=20)
plt.ylabel('columns', size=20)
plt.show()
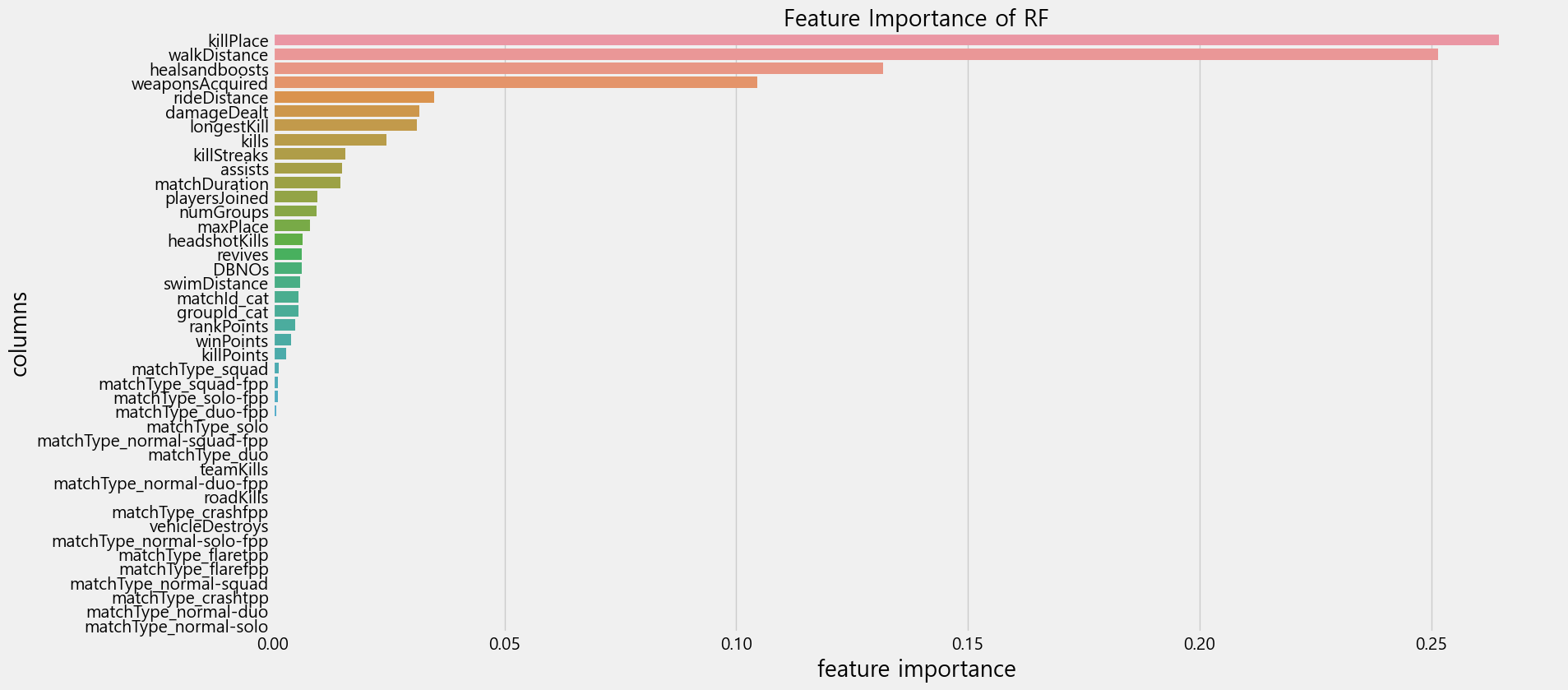
โก RF Model 2
- ํ๋ผ๋ฏธํฐ ์กฐ์ : n_estimators ๊ฐ 50 -> 80
## ํ๋ผ๋ฏธํฐ ์กฐ์ ํ ํ์ต
rf2 = RandomForestRegressor(n_estimators=80,
min_samples_leaf=3,
max_features='sqrt',
n_jobs=-1)
rf2.fit(X_train, y_train)
print_score(rf2)
# ์ถ๋ ฅ ๊ฒฐ๊ณผ
['mae train:', 0.04123858030265405, 'mae val:', 0.06211411133651147]
## Basic model์ ๋ณ์์ค์๋ ํ์ธ
rf_feature_importance2 = pd.DataFrame(rf2.feature_importances_, X_train.columns, columns=['Feature Importance'])
# ๋ณ์์ค์๋ ์์๋ก ์ค๋ฆ์ฐจ์ ์ ๋ ฌ
rf_feature_importance2 = rf_feature_importance2.sort_values('Feature Importance', ascending=False)
# ๋ณ์์ค์๋ ์๊ฐํ
plt.figure(figsize=(18,9))
sns.barplot(x='Feature Importance', y=rf_feature_importance2.index, orient='h', data=rf_feature_importance2)
plt.title("Feature Importance of RF", size=20)
plt.xticks(size=15)
plt.yticks(size=15)
plt.xlabel('feature importance', size=20)
plt.ylabel('columns', size=20)
plt.show()
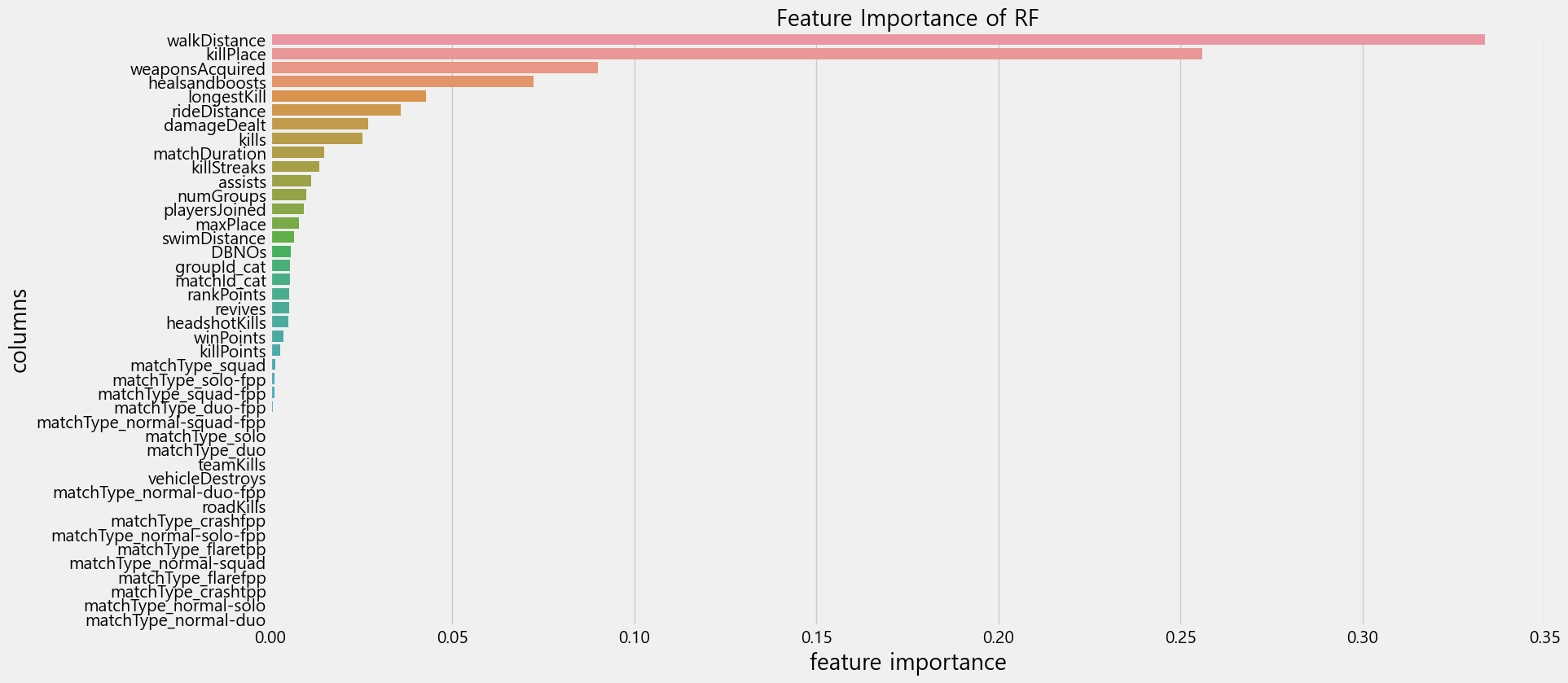
โก ์๊ด๊ด๊ณ
## Feature Importance > 0.05์ธ ๋ณ์๋ค๋ง ์ถ์ถ
df_keep = df[rf_feature_importance2[rf_feature_importance2['Feature Importance'] > 0.05].index].copy()
X_train, X_valid = split_vals(df_keep, n_trn)
## ํํธ๋งต์ผ๋ก ์๊ด๊ด๊ณ ํ์ธ
corr = df_keep.corr()
plt.figure(figsize=(10, 7))
sns.heatmap(corr, cmap="Greens", annot=True, linewidths=0.5, fmt=".3f", cbar = True)
plt.show()
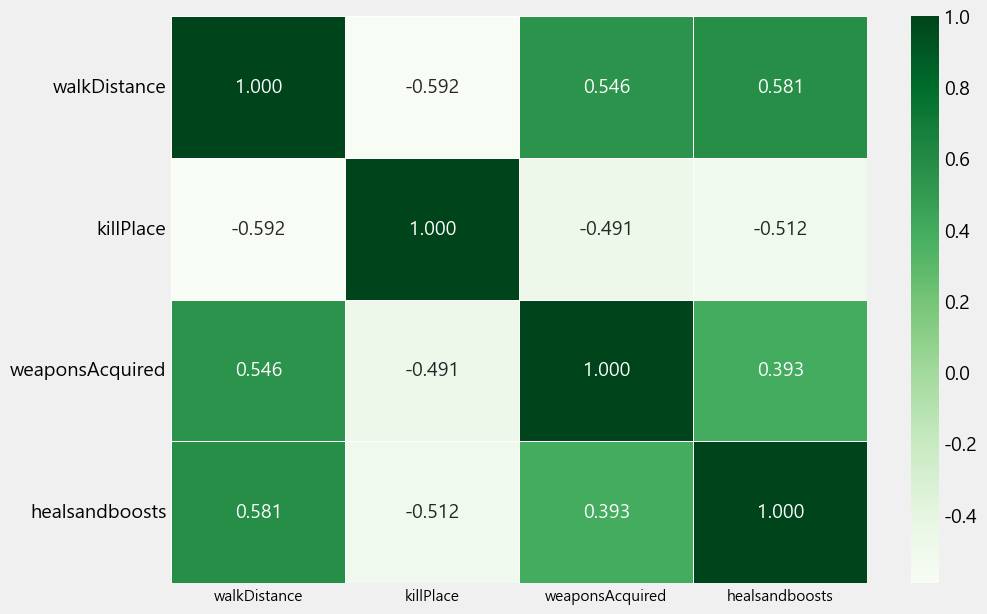
(6) ์ต์ข RF Model
## train, valid data ๋ถ๋ฆฌ
val_perc_full = 0.2
n_valid_full = int(val_perc_full * len(train))
n_trn_full = len(train) - n_valid_full
# X, y ๋ถ๋ฆฌ
y = train['winPlacePerc']
df_full = train.drop('winPlacePerc', axis=1)
# df_full = df_full[to_keep]
# Split
X_train, X_valid = split_vals(df_full, n_trn_full)
y_train, y_valid = split_vals(y, n_trn_full)
# ํ์ธ
print('train:', X_train.shape, 'target:', y_train.shape, 'validation:', X_valid.shape)
# ์ถ๋ ฅ ๊ฒฐ๊ณผ
train: (3556020, 42) target: (3556020,) validation: (889004, 42)
## ์ต์ข
RF Model ํ์ต
rf_final = RandomForestRegressor(n_estimators=80,
min_samples_leaf=3,
max_features='sqrt',
n_jobs=-1)
rf_final.fit(X_train, y_train)
print_score(rf_final)
# ์ถ๋ ฅ ๊ฒฐ๊ณผ
['mae train:', 0.0394811623200244, 'mae val:', 0.058680613710969526]
์ฐธ๊ณ ์๋ฃ
Kaggle | PUBG Data Exploration + RF (+ Funny GIFs)
๋ถ์ ์ฝ๋
'Study > Data Analysis' ์นดํ ๊ณ ๋ฆฌ์ ๋ค๋ฅธ ๊ธ
[Dacon/python] ์ ์ฃผ๋ ๋๋ก ๊ตํต๋ ์์ธก ํ๋ก์ ํธ (0) | 2023.08.24 |
---|---|
[kaggle/python] House Price prediction (0) | 2023.08.24 |
[kaggle/python] House Price exploration (0) | 2023.08.23 |
[kaggle/python] titanic ์์กด ์ฌ๋ถ ์์ธก (0) | 2023.08.23 |